Quick start guide¶
This packages implements methods described in the paper:
Exact decoding of the sequentially Markov coalescent
Caleb Ki, Jonathan Terhorst
bioRxiv 2020.09.21.307355; doi:10.1101/2020.09.21.307355
The methods are implemented in a Python package called xsmc
. The package has two main uses: drawing exact samples from the posterior distribution, and fast estimation of the maximum a posteriori (MAP) path. Convenience methods are also included for plotting.
We will demonstrate how the method works on simulated data using msprime
.
[3]:
def simulate(recombination_rate, length, sample_size=2):
return msp.simulate(
sample_size=sample_size,
mutation_rate=1e-8,
recombination_rate=recombination_rate,
Ne=1e4,
length=length,
random_seed=np.random.randint(1, int(4e9)),
demographic_events=[
msp.PopulationParametersChange(time=0.0, initial_size=1e5),
msp.PopulationParametersChange(time=1000.0, initial_size=1e4),
],
)
All methods are accessed by creating an instance of the xsmc.XSMC
class. The class has three required arguments:
ts
, atskit.TreeSequence
instance containing the data.focal
, the node id of the focal haplotype ints
.panel
, a list of node ids ints
which are potential targets forfocal
to copy from.
First, we focus on the simplest type of analysis, where we have two chromosomes sampled from a singled diploid individual.
[4]:
from xsmc import XSMC, Segmentation
data = simulate(recombination_rate=1e-9, length=1e6, sample_size=2)
xs = XSMC(data, focal=0, panel=[1], rho_over_theta=0.1)
Exact sampling¶
To sample paths from the posterior, use the XSMC.sample()
.
[5]:
paths = xs.sample(k=100, seed=1)
The returned object is a list of length k
of Segmentation
objects. These objects can be easily plotted to visualize the posterior:
[6]:
for p in paths:
p.draw(alpha=0.1)
plt.yscale("log")
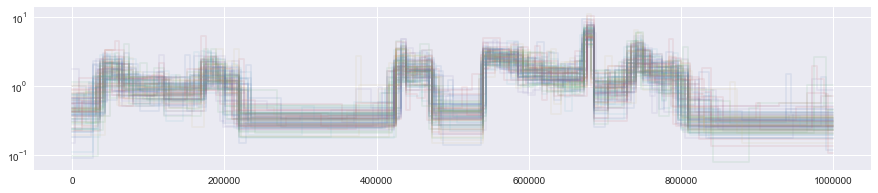
To compare the posterior with the truth, we can use built-in convenience methods to turn data into a Segmentation
object and plot it.
[7]:
truth = Segmentation.from_ts(data, focal=0, panel=[1])
truth.draw()
plt.yscale("log")
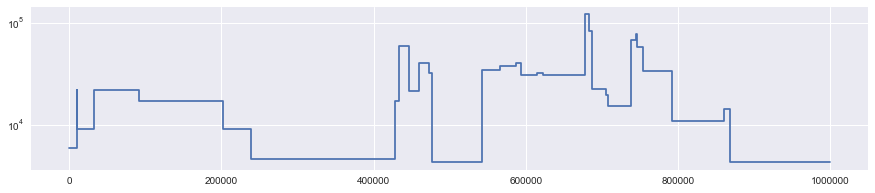
Rescaling the output¶
You may notice that the \(y\) scale of the above plots is different. By default, Segmentation
s returned by XSMC
are in units of coalescent time. This matches the scaling of the Li & Durbin’s PSMC.
To convert these times to generations, you must know the biological mutation rate \(\mu\), and then estimate \(N_0\) by the formula \(N_0 = \theta / (4 \mu)\):
[8]:
N0 = xs.theta / (4 * 1e-8)
Then, you can convert time to generations by multiplying by \(2N_0\).
[9]:
for p in paths:
p.rescale(2 * N0).draw(
color="tab:blue", alpha=0.1
) # divide by the mutation rate used in the simulation, convert to haploid
truth.draw(color="tab:red")
plt.yscale("log")
# plt.ylim(0, 1e4)
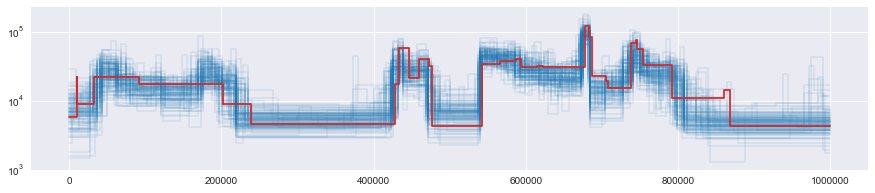
Computing functionals of the posterior¶
The Segmentation.to_pp()
method can be used to get access to the raw stepwise function it represents (returned as a piecewise polynomial, whence the name). By calling this method on paths returned from XSMC.sample_paths()
, this you can compute functionals of the posterior distribution.
[10]:
x = np.linspace(0, data.get_sequence_length(), 1000)
y = np.array([p.to_pp()(x) for p in paths])
q = (
np.quantile(y, [0.25, 0.5, 0.75], axis=0) * 2 * N0
) # faster than calling .rescale() on each path
plt.plot(x, q[1])
plt.fill_between(x, q[0], q[2], alpha=0.3)
truth.draw()
plt.yscale("log")
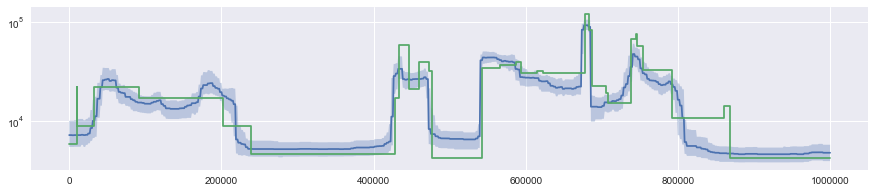
Multiple samples¶
We can analyze multiple samples using a “trunk approximation” described in the paper. In this case, the returned segmentation approximates the earliest time to coalescence with any member of the panel.
[11]:
data = simulate(recombination_rate=1e-8, length=1e6, sample_size=20)
focal = 0
panel = list(range(1, 20))
xs = XSMC(data, focal=focal, panel=panel, rho_over_theta=1.0)
paths = xs.sample(k=100, seed=1)
[12]:
N0 = xs.theta / (4 * 1e-8)
for p in paths:
p.rescale(2 * N0).draw(color="tab:blue", alpha=0.05)
truth = Segmentation.from_ts(data, focal=focal, panel=panel)
truth.draw(color="tab:red")
plt.yscale("log")
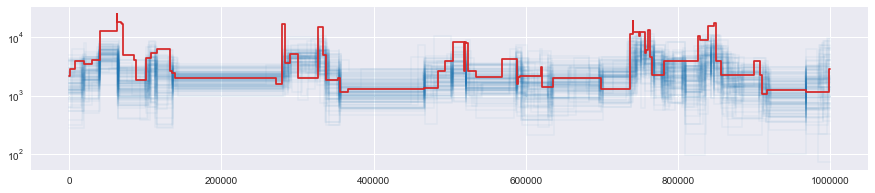
Viterbi Decoding¶
The other function of the XSMC
class is to compute the Viterbi/most probable path under the posterior:
[13]:
data = simulate(recombination_rate=1e-9, length=10e6, sample_size=10)
xs = XSMC(data, 0, [1, 2], rho_over_theta=1e-9 / 1.4e-8)
N0 = xs.theta / (4 * 1e-8)
map_path = xs.viterbi()
map_path.rescale(2 * N0).draw(label="viterbi")
Segmentation.from_ts(data, 0, [1, 2]).draw(label="truth")
plt.yscale("log")
plt.legend()
[13]:
<matplotlib.legend.Legend at 0x7f0493c47190>
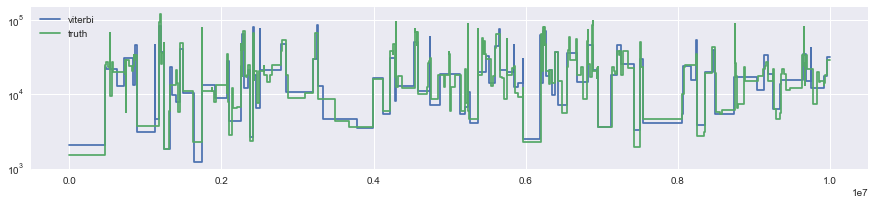
Note that each path also includes a haplotype component which is not plotted:
[14]:
map_path.segments[0]
[14]:
Segment(hap=2, interval=array([ 0, 476205]), height=0.08770837623933274, mutations=21)
This says that the first segment in the MAP path had height 0.09 (in coalecent units) and coalesced with panel haplotype 2.
[ ]: